Procurement Research at the IRS
by Shanna Webbers and Andrea Kadish
Data analysis is not new within federal procurement. However, at the nexus of computer science, statistics, and business knowledge, data scientists are using new techniques to bring operational analytics—the use of data analytics to drive real-time impact within an operational environment[i]—to the Internal Revenue Service (IRS) Office of the Chief Procurement Officer (Procurement). At the heart of the effort is the newly created Data Analytics and Technology (DAT) Division. Our team built a novel procurement research group of procurement practitioners, university professors, and students through a partnership with Data and Analytic Solutions, Inc., a woman-owned small business in Fairfax, Virginia. The group is merging analytics with emerging technologies to identify performance baselines from which to find patterns to improve processes and make predictions during times of uncertainty. What makes the IRS Procurement approach unique is that the digital transformation is not occurring within an information technology environment; rather, the organization is demonstrating that federal procurement organizations are well-suited for a culture of continuous learning and experimentation that will encourage intrapreneurs[ii] to harness the organization’s big data and bring innovative, actionable, and timely insights.
The Business Case
Shanna Webbers, IRS chief procurement officer, set expectations high when planning a recent multi-faceted transformation of IRS Procurement to power an improved business model, efficient and effective operations, and enhanced customer experience. Webbers wanted procurement professionals to focus on areas requiring critical thinking and high-level engagement and invest in their overall professional development. Both goals might improve recruitment and retention for positions identified by the U.S. Office of Personnel Management (OPM) as having a a severe shortage of candidates. Moreover, like most federal procurement organizations, the organization was facing challenges, such as s consistently increasing workload with nearly a third of its workforce eligible to retire within two years. To solve the challenges, our team needed to turn innovation theory into a reality.
We paired two of the organization’s greatest resources—intrapreneurs and federal procurement data. Intrapreneurs are employees who act like entrepreneurs within an organization, taking the initiative—and oftentimes risk—to pursue innovative products and services. In this case, we wanted our staff members to apply data analytics and emerging technologies to existing challenges. We recognized federal data as being “a valuable national resource,” as noted in the OPEN Government Data Act. Andrea Kadish, an intrapreneur who established the category management program within IRS Procurement, was selected to drive this effort as the first director of the DAT Division. Kadish used a lean startup model and began immediately delivering products using iterative development. For example, our dashboard team employed standard analysis methods—such as monitoring, slicing and dicing, and anomaly detection—to build custom procurement dashboards in weekly sprints. The goal is to establish a “common operating picture,” a term borrowed from the military to mean an integrated representation of activities across the organization to achieve situational awareness and collaboration.[iii] Dashboard development is a significant accomplishment, yet we recognized there is more to be done.
Federal procurement data can be characterized as “big data” because of its high volume, variety, and velocity (the speed with which it is generated, distributed, and collected).[iv] To make the best use of this data, we conducted a preliminary review of use cases possible through advanced data analytics: predictive analytics used for spend forecasts to drive more effective workload distribution or better acquisition strategies, estimate needed staffing levels, and predict supply chain challenges. Clustering techniques can strengthen market research by finding groupings of possible vendors, identifying historical price variances of like purchases, and identifying opportunities for requirements consolidation. Text analytics often combine statistical techniques and Natural Language Processing (NLP), which is a field of artificial intelligence that enables computers to analyze and understand human language, to derive insights from unstructured data, such as the “contract description” field in contract writing systems or entire documents, such as performance work statements. The uses cases associated with text analytics include procurement fraud detection, requirement, vendor, and product similarity evaluation.
Advanced data analytics techniques would enable IRS Procurement to advance as a value-enhancing business function with improved operational outcomes and reduced lead time. Further, data analyses can multiply their value as they are repurposed for new use cases. Similarly, advanced data analytics within IRS Procurement would support innovation within other federal agencies and the public because the IRS was interested in sharing code on Data.gov as envisioned by the OPEN Government Data Act.
The Investments
The DAT Division began its advanced data analytics efforts in the summer of 2020 through two Coding It Forward, Civic Digital Fellowship[v] projects. One project evaluated the ability to use machine learning (a form of artificial intelligence that allows computers to become more accurate at predicting outcomes without being explicitly programmed)[vi] to predict award date based on a set of variables, such as workload proportion, approval month, and days until fiscal year-end. The other project used machine learning to classify bridge contracts, which can be difficult to quantify. After developing these initial models, the team anticipated the need for more data, feature engineering, model tuning, and exploration of other models.
With these early insights, we contracted with Data and Analytic Solutions, Inc., to launch procurement research on behalf of IRS Procurement. We used the vision of the governmentwide Frictionless Acquisition initiative to guide the overall research direction. The initiative envisions procurement organizations as being strategic business partners in the achievement of their agencies’ mission. It also asserts that public-sector procurement organizations can use emerging technologies and best practices to improve Procurement Administration Lead Time (PALT), which was recently defined by the Office of Federal Procurement Policy in the U.S. Office of Management and Budget as the period between solicitation and award.[vii] We launched a study of contract award time with three areas of focus: (1) a model that identifies significant PALT drivers, (2) a model that predicts contract award dates, and (3) NLP of the “contract description” field in usaspending.gov data that provides contextual information about contracts. The study is based on recent governmentwide records for approximately 5.5 million contracts, potentially making it the largest-ever federal government PALT study. The research team considered data availability, risk, and organizational value prior to pursuing the projects described below.
PALT Explanatory Model
Our PALT Explanatory Model addresses the customer concern that the procurement process “takes too long” by identifying the factors that influence award time. It uses a Random Forest regression machine learning model because it is easier to understand and use and features high accuracy. The regression algorithm measures the relationship between the dependent variable (i.e., PALT) and independent variables likely to affect PALT (e.g., days remaining in the fiscal year, obligation value, number of offers, North American Industry Classification System (NAICS) code, solicitation procedures, set-aside type, contract vehicle, and contract type). It associated a quantity of PALT days with specific variables.
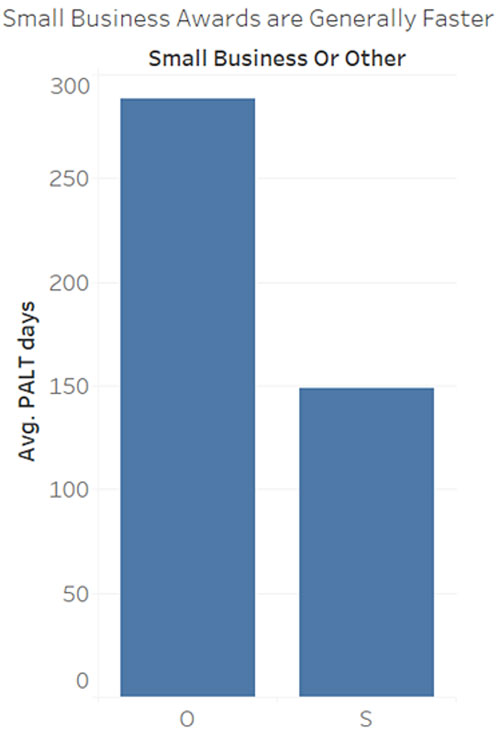
A contracting officer could use the model findings to advise the customer how certain acquisition approaches could change award time. For instance, awards to small businesses are generally faster than those to other businesses, as shown in Figure 1. Or, as shown in Figure 2, the average PALT for certain contract types varies.

Contract Award Date Forecasting Model
The Contract Award Date Forecasting Model predicts the date of contract award based on specific requirement features. The research team built the model with machine learning to predict the contract award timeline from requisition approval to award. We used a Random Forest regression approach, which revealed that the strongest indicators of lead time were contract specialist workload, functional area, and days remaining until the end of the fiscal year. The model predicts award dates within 30 days with 86 percent accuracy, within the same month with 61 percent accuracy, and within seven days with 44 percent accuracy.
To make the model accessible to users, the team built a web interface for users to input features of their requisitions and obtain an estimated award date. The features include fiscal year, agency, date the requisition reached IRS Procurement, organizational information for the contracting specialist, obligated dollars, funding business unit, and program information. Four additional features focused on workload and time: count of days until the end of the fiscal year; the total number of requisitions completed by the contracting specialist within the last 90 days; the current total of requisitions assigned to the contracting specialist; and a calculation of the contracting specialist’s workload as a proportion of the specialist’s total amount of work in the last 90 days. The output of the web application is a custom prediction for award date based on selected features, as shown in Figure 3.
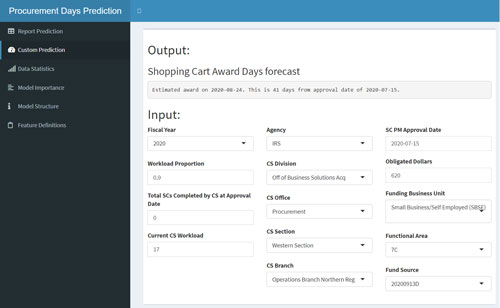
The organization plans to use this research to refine deadlines for submission of acquisition packages. The organization executes between 10,000 and 12,000 actions per year, totaling more than $2.5 billion in obligations. The lead time for this significant workload must allow enough time for acquisition planning and creation of innovative business strategies with a strong focus on fiscal management and accountability. IRS Procurement currently sets deadlines based on the dollar value for the entire period of performance. However, additional time must be added for complex acquisitions or to accommodate a successful transition—meaning that setting deadlines solely on total contract value may not allow for enough time for efficient and effective contract awards.
Text Analytics
Text analytics solutions offer some of the most exciting insights for federal procurement organizations. Most analytics within federal procurement have focused on structured data, which is neatly organized data with a defined length and format. Within the procurement environment, structured data is exemplified by the approximately 150 data elements per contract reported to Federal Procurement Data System (FPDS-NG), such as Taxpayer Identification Number, contractor name and address, and socioeconomic information. One exception in the FPDS-NG is the contract description, which is subject to a character limit, but the content is based on the judgment of the procurement professional. There are additional structured data examples within specific contract writing systems. However, many insights have been inaccessible from the significant documentation within each contract file, such as statements of work that include unstructured and semi-structured data. Text analytics offer the solution for analyzing this previously inaccessible data.
The initial use case looked at the award description field in the usaspending.gov dataset to determine whether awards with similar contract descriptions will have similar PALT. Before a computer could analyze the text, the team prepared the text for a computer to read. The data went through text pre-processing (where the text was simplified by removing common words and reducing words to their stems) and vectorization (where the word stems were converted to a set of real numbers—a vector—and counted). A generalized linear model evaluated the importance of each word stem. The team applied an NLP algorithm called Latent Dirichlet Allocation, a type of topic modeling that identifies a set of topics in a document based on word occurrences.
Following the successful initial use case, the team considered NLP research applications in two additional areas: category management and market research.
- Category Management
The federal category management initiative positions the federal government to buy smarter and more like a single enterprise.[viii] The approach encourages requirements and contract consolidation. Current category management data analysis focuses significantly on Product Service Codes (PSC) and NAICS codes to determine whether a requirement could be placed on a governmentwide acquisition contract. Some contracting officers have reported concerns about the accuracy of this approach when more than one code can apply to a contract. Code selection can be difficult because hundreds of codes are available for selection, some having similar descriptions. The codes must be regularly updated to keep up with products and services purchased by the federal government. In addition, the code selected represents the predominant code; for example, if 51 percent of a contract is consulting and 49 percent is training, only the consulting code will be submitted.
Text analytics could supplement the current code-based approach for category management by offering more granularity available through the award description. For example, the research team considered the challenge of managing software spend. We decided to locate contract awards potentially related to a name-brand cybersecurity software using an NLP algorithm. The model’s results identified a list of contracts that might be related to the name-brand software, organized by a similarity score.
- Market Research
Text analytics offer opportunities to address market research challenges. Procurement professionals rely heavily on vendor capability statements and marketing materials. It can be difficult to assess whether a vendor has relevant experience performing similar work for federal agencies or other clients. A contracting officer may also struggle to identify additional vendors providing highly specific products or services sought by agency technical experts.
An NLP algorithm could allow procurement professionals to search millions of contract records governmentwide. We could comb contract descriptions for the most meaningful words, permitting identification of vendors with similar products or services. Likewise, NLP could identify alternatives to brand-name products recommended by customers.
Data Strategy Training
For IRS Procurement to succeed in its goal to become a data-driven organization, its procurement professionals must be data literate. Thus, the keystone of our procurement research was a series of group trainings relating data-science strategy to the procurement profession. In addition, a data-advocate program was launched for one-on-one, hands-on training for IRS Procurement staff members outside of DAT to study use case development and creation of data visualizations and associated storytelling. The training is the start of a center-of-excellence approach, where the DAT Division delivers, analyzes, and empowers peer divisions to use data strategically.
The Way Ahead
IRS Procurement has taken a significant step in leveraging data as a strategic asset. Through procurement research, we are building a culture that values data and understands how trends and predictions offered through data analytics and emerging technology can bring organizational change. We have already begun solving some of the most difficult challenges, and our team is excited to bring innovative solutions for our customers carrying out the mission of administering the nation’s tax code.
Shanna Webbers
- Chief Procurement Officer for the Internal Revenue Service (IRS).
- Responsible for the IT contractual commitments of Treasury Departmental Offices and the Bureau of Engraving and Printing.
- Served as Acting Senior Procurement Executive for the Department of the Treasury
- 25 years’ experience working in various organizations throughout the Department of Defense, including the Office of the Secretary of Defense, Secretary of the Navy, U.S. Marine Corps and the Defense Logistics Agency.
- 2014 graduate of the Senior Executive Service
Andrea Kadish
- Led the startup of a division within the IRS Office of the Chief Procurement Officer focusing on data analytics, research, and emerging technologies.
- Leads the startup of a process automation center of excellence and an enterprise risk management program at the Centers for Medicare & Medicaid Services (CMS).
- Holds a Juris Doctorate from the Sandra Day O’Connor College of Law at Arizona State University
- Alumnus of the Fulbright Program,
- Former U.S. Naval Officer
Endnotes
1 Paulevich, S. V., Nikolaevich, K. A., & Mikhailovna, P. Y. (2019). “Change from economic analysis to operational analytics and corporate analysis in innovative entrepreneurship.” Academy of Entrepreneurship Journal, 25, 1-5.
2 Pinchot, G. III (1985), Intrapreneuring, Harper & Row, New York, NY.
3 Joint Chiefs of Staff, Doctrine for Joint Operations, Joint Pub 3-0, (Washington, DC: Sep 2001), GL-7.
4 Bydon, M., Schirmer, C.M., Oermann, E.K., Kitagawa, R.S., Pouratian, N., Davies, J., Sharan, A., and Chambless, L.B. (2020). “Big Data Defined: A Practical Review for Neurosurgeons,” World Neurosurgery, 133, e842-e849.
5 Coding It Forward, “Civic Digital Fellowship,” accessed April 21, 2021, https://www.codingitforward.com/civic-digital-fellowship.
6 Mitchell, T.M. (2006). “The Discipline of Machine Learning,” CMU-ML-06-108, Carnegie Mellon University, Pittsburgh: PA.
7 Wooten, M.E., “Reducing procurement administrative lead time using modern business
practices,” accessed April 21, 2021, https://www.whitehouse.gov/wp-content/uploads/2021/01/OFPPPALTMemorandum-01-14-2021.pdf.
8 Office of Management and Budget, Memorandum for the Heads of Executive Departments and Agencies: Category Management: Making Smarter Use of Common Contract Solutions and Practices, OMB Memorandum M-19-13 (Washington, D.C.: Mar. 20, 2019).